Small area quantile estimation via spline regression and empirical likelihood
Section 6. Empirical application
We
now illustrate the proposed estimators based on the data set Survey of
Labour and Income Dynamics (SLID) provided by Statistics Canada (2014)
downloaded from University of British Columbia library data centre. The data
contain 147 variables and 47,705 sample units. We are grateful to Statistics
Canada for making the data set available, but we do not address the original
goal of the survey here. Instead, we use it as a superpopulation to study the
effectiveness of the proposed small area quantile estimator.
In
this study, we singled out 9 of the 147 variables. They are ttin, gender, spouse, edu, age, yrx, tweek, jobdur
and tpaid, standing respectively for:
total income, gender, whether living with the spouse, the highest level of
education, age, years of experience, number of weeks employed, education level,
months of duration of current job and total hours paid at this job. After
removing units containing missing values in these 9 variables as well as those
with ttin
we obtained a data set containing 28,302
sample units. The covariates power means at the population level are still
calculated based on all available observations. We created 28 sub-populations
(namely small areas) labeled as
based on gender-spouse-edu combinations. Here
denotes education level and
denote male living with the spouse, female
living with spouse, male not living with spouse and female not living with
spouse respectively. The education levels are given as follows.
kl Highest education level
1 No more than 10 years elementary and secondary school
2 11-13 years of elementary and secondary school (but did not graduate)
3 Graduated high school
4 Sorne university or non-university postsecondary with no certificate
5 Non-university postsecondary or university certificate below Bachelor's
6 Bachelor's degree
7 University certificate above Bachelor's
We
regarded
(ttin)
as the response variable and fitted linear and
additive non-parametric regressions with respect to other 5 variables. Based on
the whole data, the adjusted R-square of the non-parametric fit is 0.482 which
is much larger than 0.370 obtained by fitting the linear regression. This
suggests that a non-parametric mixed model is a good choice. Figure 6.1
shows the fitted curves of log ttin
with respect to these two covariates. Also, the
R-square is as high as 0.483 even if the model includes only covariates age
and tpaid and a random effect. These
exploratory analyses prompt us to use only these two covariates in our
simulation. We carried the simulation with sample sizes
and 1,000. To make sampling proportions in
small areas close to their sizes, we let
with
generated from the multinomial distribution
with

Description for Figure 6.1
Figure showing two graphs of the curves of log(ttin) for age and tpaid. Log(ttin) is on the y-axis, ranging from -1.5 to 0.5. For the first graph, age is on the x-axis, ranging from below 20 to 70 years old. Log(ttin) increases strongly with age, then reaches a plateau slightly above 0 for age around 30 to 60, and then starts to increase again. For the second graph, tpaid is on the x-axis, ranging from 0 to 5,000. Log(ttin) shows a strong increase (up to almost 0.5) for tpaid = 0 to about tpaid = 2,000, before slowly decreasing to about log(ttin) = 0.
The
simulated AMSE of 10 estimators based on 1,000 repetitions are reported in
Table 6.1. We first notice that both our PEL estimators outperform the
other estimators, in general, indicating the advantage of our non-parametric
DRM based small area estimation technique. The PEL1 compared to PEL2 has the
lower AMSE for 5%, 25%, and 50% quantiles, but slightly higher AMSE for 75% and
95% quantiles indicating the heteroscedasticity of data is not serious.
Regardless the PEL estimators, we notice the LEL estimators outperform other
estimators for 5% quantile, and have similar performance for other quantiles.
Increasing the sample size reduces the AMSE of all estimators. Clearly, it is
hard to estimate the 5% quantile with a good precision because the data are
skewed toward the left so there are few observations for estimating the lower
quantiles. Interestingly, LEL1 is not affected as much by the skewness. We feel
that the kernel smoothing step (3.7) is helpful here. Without this smoothing
step, LEL1 would perform much worse. Unreported simulations show that the ABIAS
of all estimators decreases in general as the sample size increases and this is
most apparent for DE.
To
check the performance of the proposed first estimator which using only
covariate average information. In Figures 6.2, we depict the 2.5%, 50%,
and 97.5% quantiles of 1,000 small area median estimates by the DE, LEL1, LEL2,
PEL1, PEL2 with sample size
with the true medians marked by dots. The
y-axis is the total income and x-axis is the education level. It is seen that
the PEL2 boxes are the shortest for most small areas.
Table 6.2
reports the bootstrap MSE estimates as well as the average ratios of bootstrap
and simulated MSEs of the small area median estimators based on
and
with sample size
The number of simulation repetition is 500
with basis function
and
We can see the estimator
has higher MSE than
and most average ratios close to one.
Table 6.1
AMSE of small area quantile estimators based on real data
Table summary
This table displays the results of AMSE of small area quantile estimators based on real data , EB0, EB1, EB2, MQ0, MQ1, MQ2, LEL1, LEL2, PEL1 and PEL2 (appearing as column headers).
|
|
EB0 |
EB1 |
EB2 |
MQ0 |
MQ1 |
MQ2 |
LEL1 |
LEL2 |
PEL1 |
PEL2 |
n = 200 |
5% |
0.784 |
0.769 |
0.901 |
0.714 |
0.763 |
0.885 |
0.245 |
0.421 |
0.242 |
0.336 |
25% |
0.107 |
0.256 |
0.488 |
0.102 |
0.261 |
0.467 |
0.115 |
0.131 |
0.097 |
0.152 |
50% |
0.080 |
0.119 |
0.236 |
0.064 |
0.116 |
0.223 |
0.076 |
0.095 |
0.056 |
0.102 |
75% |
0.122 |
0.100 |
0.142 |
0.085 |
0.102 |
0.138 |
0.085 |
0.076 |
0.069 |
0.068 |
95% |
0.233 |
0.190 |
0.280 |
0.141 |
0.138 |
0.266 |
0.217 |
0.179 |
0.117 |
0.096 |
n = 500 |
5% |
0.793 |
0.603 |
0.826 |
0.710 |
0.579 |
0.805 |
0.173 |
0.345 |
0.210 |
0.301 |
25% |
0.072 |
0.110 |
0.207 |
0.076 |
0.119 |
0.197 |
0.069 |
0.127 |
0.063 |
0.091 |
50% |
0.049 |
0.050 |
0.074 |
0.036 |
0.050 |
0.072 |
0.053 |
0.076 |
0.040 |
0.043 |
75% |
0.108 |
0.044 |
0.060 |
0.055 |
0.046 |
0.058 |
0.054 |
0.047 |
0.046 |
0.043 |
95% |
0.257 |
0.128 |
0.152 |
0.109 |
0.058 |
0.148 |
0.138 |
0.125 |
0.086 |
0.077 |
n = 1,000 |
5% |
0.792 |
0.397 |
0.542 |
0.706 |
0.377 |
0.528 |
0.078 |
0.130 |
0.095 |
0.144 |
25% |
0.054 |
0.056 |
0.098 |
0.066 |
0.067 |
0.095 |
0.041 |
0.043 |
0.038 |
0.056 |
50% |
0.034 |
0.026 |
0.032 |
0.027 |
0.026 |
0.031 |
0.019 |
0.028 |
0.018 |
0.024 |
75% |
0.102 |
0.024 |
0.030 |
0.043 |
0.026 |
0.030 |
0.037 |
0.033 |
0.019 |
0.023 |
95% |
0.270 |
0.088 |
0.090 |
0.095 |
0.114 |
0.090 |
0.074 |
0.067 |
0.053 |
0.057 |
Table 6.2
Bootstrap MSE estimates and average ratios of the estimated and simulated MSEs
Table summary
This table displays the results of Bootstrap MSE estimates and average ratios of the estimated and simulated MSEs. The information is grouped by (appearing as row headers), (équation) (appearing as column headers).
|
|
|
5% |
25% |
50% |
75% |
95% |
5% |
25% |
50% |
75% |
95% |
MSE |
0.542 |
0.196 |
0.117 |
0.098 |
0.165 |
0.204 |
0.093 |
0.068 |
0.062 |
0.102 |
Ratio |
0.843 |
0.959 |
1.014 |
0.988 |
0.871 |
0.969 |
0.994 |
1.003 |
0.996 |
0.975 |
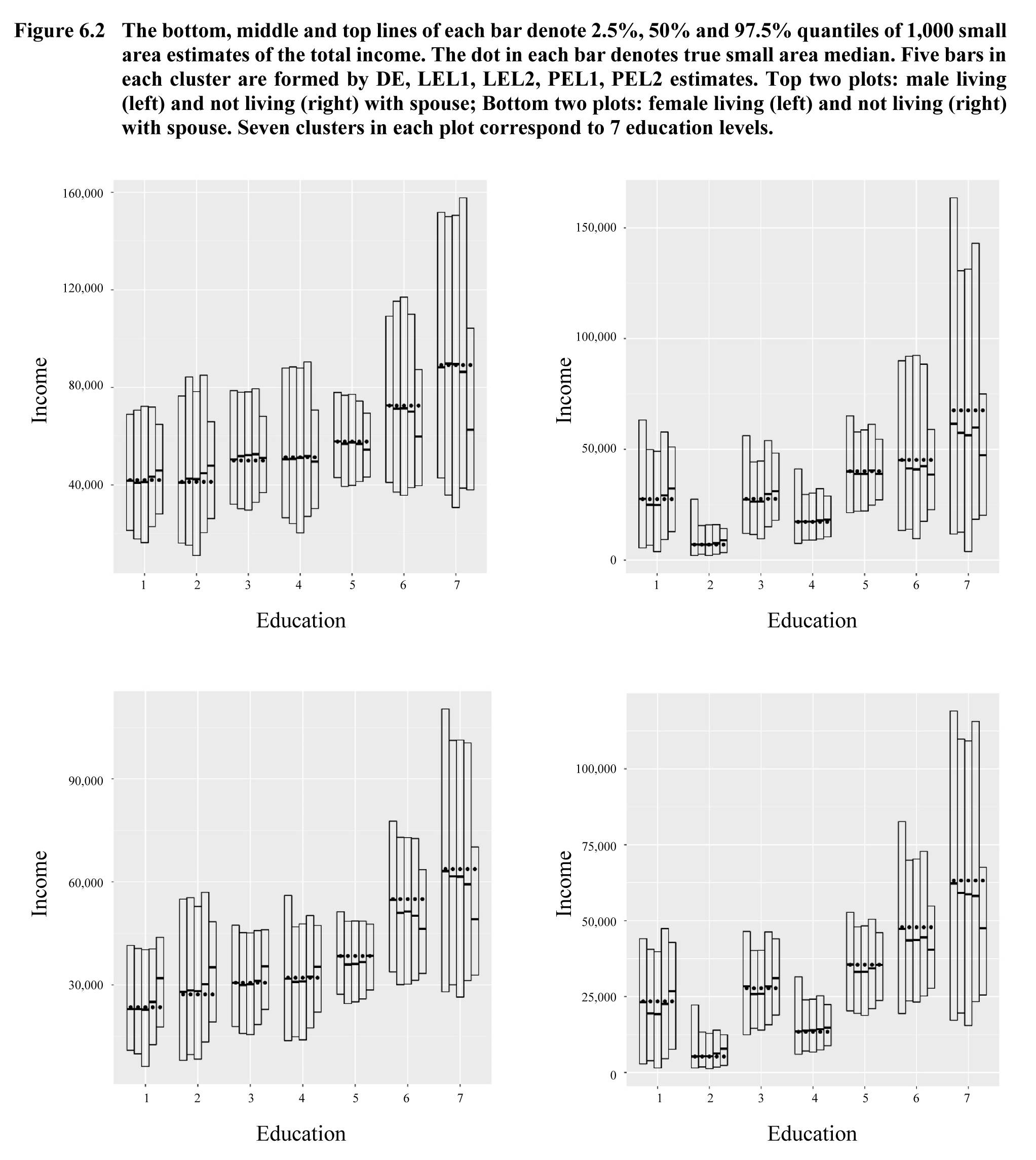
Description for Figure 6.2
Figure illustrating the 2.5%, 50% and 97.5% quantiles of 1,000 small area median estimates of total income for five estimators: DE, LEL1, LEL2, PEL1 and PEL2. There are four graphs: male living (a) and not living (b) with spouse and female living (c) and not living (d) with spouse. Income is on y-axis, ranging from 0 to 160,000, from 0 to 150,000, from 0 to a little over 105,000 and from 0 to a little above 115,000 for graphs (a) to (d) respectively. Education, divided in 7 clusters, is on x-axis. For each education cluster, quantiles obtained with the above five estimators are depicted by a vertical bar. The bottom, middle and top lines of each bar denote 2.5%, 50% and 97.5% quantiles. The true median is also depicted in each bar. For each graph, the largest and highest bars correspond to education cluster 7. For graphs (b) and (d), the shortest and lowest bars correspond to education cluster 2. For graphs (a) and (c), the income quantiles increase when education increases. The PEL2 bars are the shortest for most cases.
ISSN : 1492-0921
Editorial policy
Survey Methodology publishes articles dealing with various aspects of statistical development relevant to a statistical agency, such as design issues in the context of practical constraints, use of different data sources and collection techniques, total survey error, survey evaluation, research in survey methodology, time series analysis, seasonal adjustment, demographic studies, data integration, estimation and data analysis methods, and general survey systems development. The emphasis is placed on the development and evaluation of specific methodologies as applied to data collection or the data themselves. All papers will be refereed. However, the authors retain full responsibility for the contents of their papers and opinions expressed are not necessarily those of the Editorial Board or of Statistics Canada.
Submission of Manuscripts
Survey Methodology is published twice a year in electronic format. Authors are invited to submit their articles in English or French in electronic form, preferably in Word to the Editor, (statcan.smj-rte.statcan@canada.ca, Statistics Canada, 150 Tunney’s Pasture Driveway, Ottawa, Ontario, Canada, K1A 0T6). For formatting instructions, please see the guidelines provided in the journal and on the web site (www.statcan.gc.ca/SurveyMethodology).
Note of appreciation
Canada owes the success of its statistical system to a long-standing partnership between Statistics Canada, the citizens of Canada, its businesses, governments and other institutions. Accurate and timely statistical information could not be produced without their continued co-operation and goodwill.
Standards of service to the public
Statistics Canada is committed to serving its clients in a prompt, reliable and courteous manner. To this end, the Agency has developed standards of service which its employees observe in serving its clients.
Copyright
Published by authority of the Minister responsible for Statistics Canada.
© Her Majesty the Queen in Right of Canada as represented by the Minister of Industry, 2019
Use of this publication is governed by the Statistics Canada Open Licence Agreement.
Catalogue No. 12-001-X
Frequency: Semi-annual
Ottawa